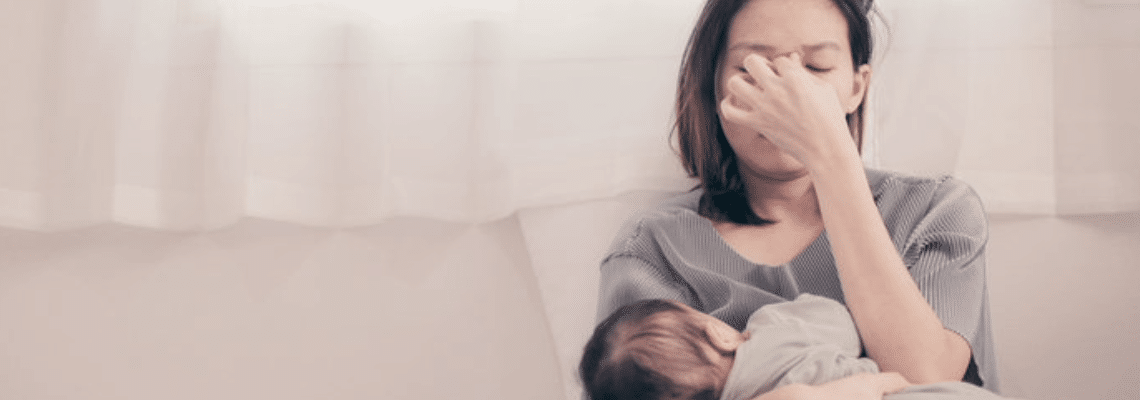
Postpartum depression (PPD) affects up to 15 percent of all women after giving birth. Early detection of high-risk patients could improve proactive psychological care. Researchers at Mass General Brigham have developed a machine learning model that can assess patients’ risk of PPD based on easily accessible clinical and demographic factors. The results, which demonstrate the model’s promising predictive capabilities, were published in the American Journal of Psychiatry.
Nearly 30 Percent of All Patients Predicted to be at High Risk Developed Postpartum Depression Within Six Months of Giving Birth
“Postpartum depression is one of the biggest challenges some parents face in the period after childbirth—a time when many are struggling with sleep deprivation, new stresses, and significant life changes,” said lead author Mark Clapp, MD, MPH, of the Department of Obstetrics and Gynecology at Massachusetts General Hospital, a founding member of the Mass General Brigham Health System. “Persistent feelings of sadness, depression, or anxiety may be more common than many people realize. Our team, led by Dr. Roy Perlis, has conducted this work to better understand which patients are at higher risk for PPD so that we can develop strategies and solutions to either prevent it or reduce its severity.”
Typically, PPD symptoms are assessed during postpartum follow-up visits, which take place 6 to 8 weeks after delivery. As a result, many parents may struggle for several weeks before receiving psychological help. To enable earlier PPD care, the researchers developed a model that requires only information available in the electronic health record (EHR) at the time of delivery, including data on demographics, medical conditions, and previous examinations. This model weights and integrates these complex variables to more accurately assess the risk of postpartum depression. To develop and validate the model, the authors used information from 29,168 pregnant patients who gave birth between 2017 and 2022 at two academic medical centers and six community hospitals in the Mass General Brigham system. In this cohort, 9 percent of patients met the study criteria for PPD in the six months after giving birth.
The researchers used health data from about half of the patients to train the model to detect postpartum depression. They then tested the model by asking it to predict PPD in the other half of the patients. The researchers found that the model was effective in ruling out postpartum depression in 90 percent of cases. The model showed promising results in terms of prediction: nearly 30 percent of all patients who were predicted to be at high risk developed depression within six months of giving birth.
Improving Mental Health of Mothers
The model was about two to three times better at predicting PPD than estimates based on the risk in the general population. In further analyses, the researchers showed that the model worked similarly well regardless of race, ethnicity, and age at delivery. The study included only individuals with no previous psychiatric diagnosis in order to determine whether the model can also predict PPD in low-risk patients and to better understand the risk factors that influence postpartum depression regardless of previous psychiatric diagnoses. Notably, Edinburgh Postnatal Depression Scale scores obtained during pregnancy improved the model’s predictive ability, suggesting that this existing tool may be useful both before and after delivery.
The researchers are currently prospectively testing the accuracy of the model—an essential step toward practical application—and are working with patients, clinicians, and stakeholders to determine how the information gained from the model can best be integrated into clinical practice. “This is an exciting step forward in the development of a predictive tool that, when combined with the expertise of clinicians, could help improve the mental health of mothers,” said Clapp. With further validation and collaboration with clinicians and patients, the researchers hope to achieve earlier detection and ultimately better mental health outcomes for postpartum patients.

